At Helm.ai, we believe strongly that Unsupervised Learning will be the way in which autonomous vehicle technology becomes more robust and scalable, and spent some time late last week fleshing out our point-of-view and facilitating discussion around it at a leading industry conference.
This year’s (virtual) Autonomous Vehicles Summit, hosted by tech news outlet The Information, took place this past Thursday and Friday, June 25 and 26. We were a corporate sponsor for the event, and we were excited to engage in discussions with other top minds in the autonomous vehicle industry.
The mix of fireside chats and expert panels featured additional insights from SMEs representing major players like Nuro, Aurora, Lyft, Wayve, Voyage, Starship Technologies, CloudTrucks, Sequoia Capital, Embark, Zoox, Pronto, Locomotion, Kodiak Robotics, Driving Joint Venture and Trucks VC.
Some topics touched upon during the two-day event addressed maximizing the efficiency of R&D, deciding which safety metrics were most appropriate for public sharing, finding the right road areas for testing and development, and replicating automated driving software across multiple urban areas.
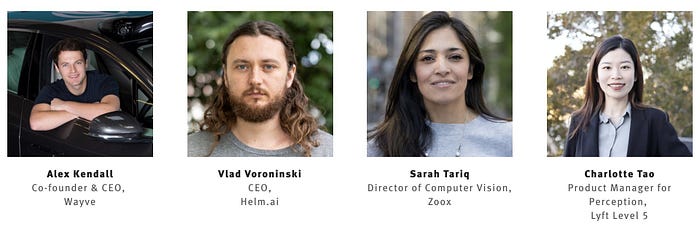
In the Sim Smarts Panel Discussion, held Thursday, June 25, at 10 a.m. PST, Helm.ai CEO Vlad Voroninski joined three other industry experts — Alex Kendall (Wayve AI), Sarah Tariq (Zoox) and Charlotte Tao (Lyft) — along with host and panel moderator Amir Efrati (The Information). During the session we fielded questions about the future viability and scalability of the methodologies and strategies used today to develop the AI software powering autonomous driving.
These traditional methods include creating and updating digital 3D roadmaps, as well as training automated driving algorithms by using images and videos manually labeled by humans … also known as Supervised Learning.
“For truly scalable technology, traditional AI methods aren’t enough to get you there” — Helm.ai CEO Vlad Voroninski
Knowing the exhaustive length of time this manual, individual labeling takes we’ve pioneered Deep Teaching, a methodology that requires no human annotation or simulation, instead letting our machine learning algorithms do the work. This reduces bottlenecks, and increases capital efficiency.
Panelist and Lyft Level 5 Self-Driving Division Product Manager Charlotte Tao seemed to agree with our assessment, admitting her colleagues have started a “separate thread” of research on unsupervised machine learning. As summarized in Mr. Efrati’s post-event commentary, Ms. Tao suggested “Lyft’s prototypes “can never” collect and label enough real-world imagery to cover all possible scenarios and objects.”
This signals a fundamental industry shift. Our “Deep Teaching” Unsupervised Learning model enables faster Deep Learning, allowing for orders-of-magnitude greater data-per-dollar spend, and more accurate results. This puts us in a trailblazing position, and we believe it will drive the future of autonomous technologies well beyond even the road. Check out our highlight reel of Vlad’s contributions during the panel below.
We would like to thank The Information for hosting the event and for inviting us to sponsor and engage in discussions. They did a tremendous job pivoting to an all-virtual format in the wake of the COVID-19 pandemic.